Traffic-Related Air Pollution (TRAP) from vehicle emissions is harmful to the environment and health (Khreis et al., 2023). Several studies have been carried out on urban traffic control techniques and other interventions to reduce vehicle emissions (see some of the works listed in Khreis et al., 2023). However, these same studies have focused on reducing the total amount of emissions, ignoring how they are dispersed in the urban environment. Thus, although they reduce emissions, they can create areas of higher concentration of emissions that violate legal limits. In addition, they can, unintentionally, relocate emissions to other areas that affect people not responsible for their generation, which can be seen as an injustice (Barnes et al., 2019).
In this project we revisit the problem of urban traffic analysis, operation, optimization, and control by integrating three simulators (see below for more details): Polaris, a traffic simulator; Autonomie, a fuel consumption and pollutant emissions simulator; and AERMOD, an atmospheric dispersion model. The integration of these three tools opens a new perspective for the analysis, optimization, and control of urban traffic systems with respect to TRAP. Instead of considering only the aggregate of emissions, it is possible to consider their dispersion. Thus, operational and control decisions can be made to prioritize, for example, areas more susceptible to pollutant concentration, homogenization of exposure to pollutants, and compliance with air quality standards, in addition to reducing the total amount of emissions.
Transportation is responsible for a quarter of global carbon dioxide emissions (Cars…, 2023). Of this amount, 45% comes from passenger vehicles, such as cars, motorcycles, and buses, and 29% from freight vehicles, such as trucks. Although Brazil contributes much less to this scenario than other nations (Ritchie et al., 2020), such as China and the USA, the national figures are also significant. Vehicle emissions are composed by several gases that are harmful to the environment and health, such as CO2, NOx, and HC, in addition to particulate matter such as PM10 and PM2.5.
The health effects are not discussed so often. However, as pointed out by Khreis et al. (2023), based on work by other authors, the health costs resulting from air pollution in 2010 in countries such as the USA, Japan, Germany and the United Kingdom were between 85 and 500 million dollars. Some consequences of air pollution are premature death, and damage to different systems, such as cardiovascular and cerebrovascular, respiratory, neurological, reproductive, etc. Also according to Khreis et al. (2023), based on data from the World Health Organization, many cities have difficulty in maintaining adequate levels of air quality.
In this context, the main objective of this research proposal is to design urban traffic control and optimization strategies for large-scale networks that improve traffic conditions, reduce traffic-related pollutant emissions, and take into account their dispersion in order to reduce the number of violations of environmental standards and unequal exposure to pollutants.
Other pollutant emitters can affect air quality, such as industry and heating systems. Initially, these sources will not be considered in the development of the work. However, if data are available, they can be included in the dispersion model and, therefore, influence control decisions. The techniques developed can also be extrapolated to other problems, such as vehicle routing and fleet allocation. Depending on weather conditions, electric vehicles, such as electric buses, could be routed or allocated to areas more susceptible to exceeding legal limits for pollutant concentrations, while combustion vehicles are routed or allocated to other areas.
Given the nature of the problem, the focus of the study will be on large-scale traffic networks where the analysis of pollutant dispersion makes more sense. Since the proposal is based on complex models, a simulation-based optimization and control approach will be adopted. Non-derivative-free optimization techniques (Conn et al., 2009, Osorio and Bierlaire, 2013) will be used.
Our development is based on the use of three tools:
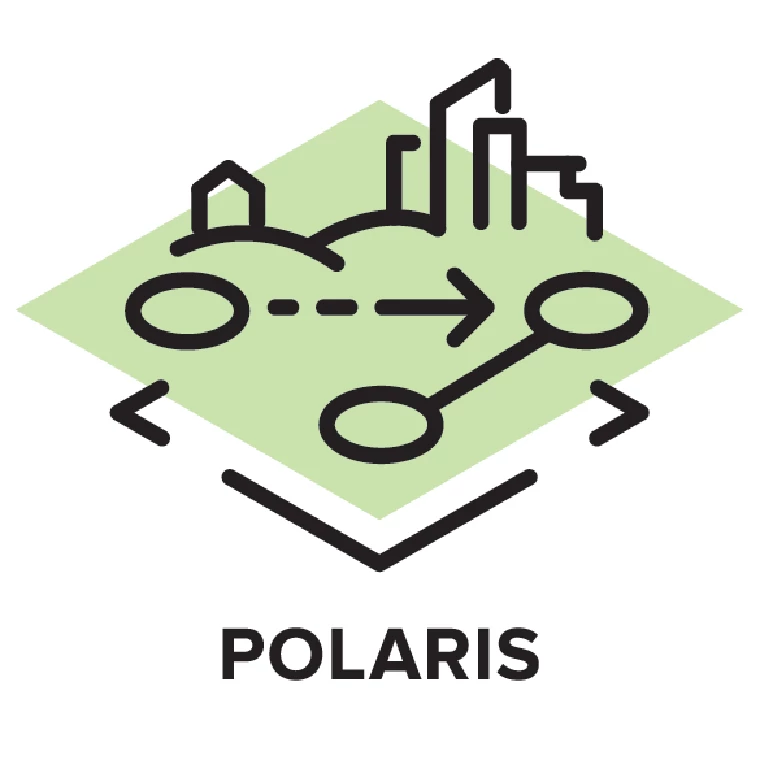
Polaris (Auld et al., 2016; Souza et al., 2019): is a high-performance mesoscopic agent-based traffic simulator developed by the Argonne National Laboratory Vehicle & Systems Mobility Group. Polaris allows travel and freight demand modeling, dynamic traffic allocation, and transportation simulation in an integrated modeling platform. In addition, traffic management can be implemented and different types of vehicles can be considered.
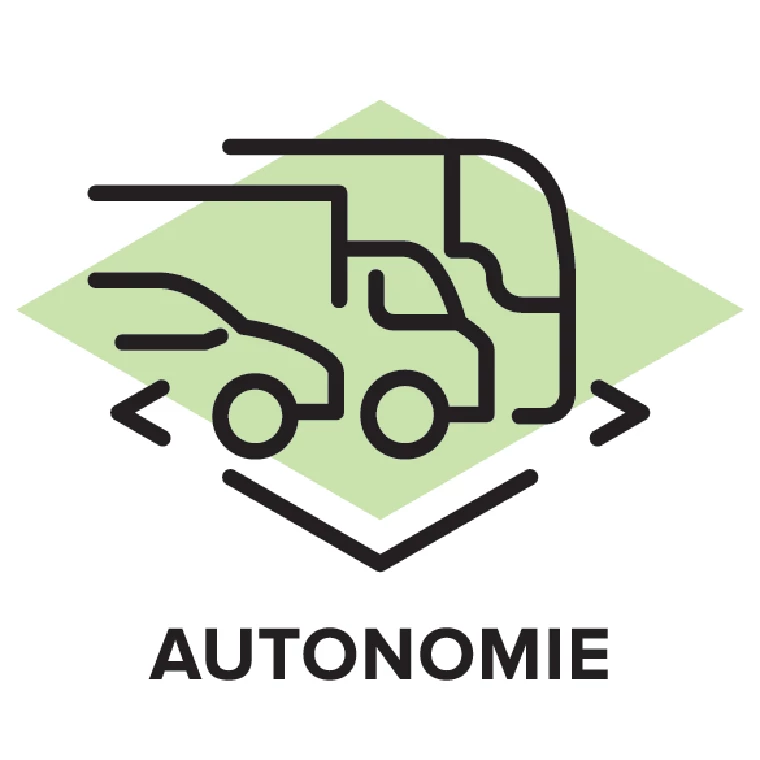
Autonomie (Kim et al., 2012): is a vehicle systems simulation tool developed by the Argonne National Laboratory Vehicle & Systems Mobility Group. This simulator allows the evaluation of energy consumption, performance and cost of different vehicle technologies, including different classes of vehicles, from light to heavy, different types of fuel, such as gasoline, diesel and ethanol, as well as electric vehicles, hybrids and variations. It receives driving cycles as input and the outputs are computed through models of the vehicle components. Emissions associated with fuel consumption are also provided from the source. That is, not only the emissions produced by the vehicle are modeled, but also the emissions produced for the extraction and production of fuel or for the generation of energy.
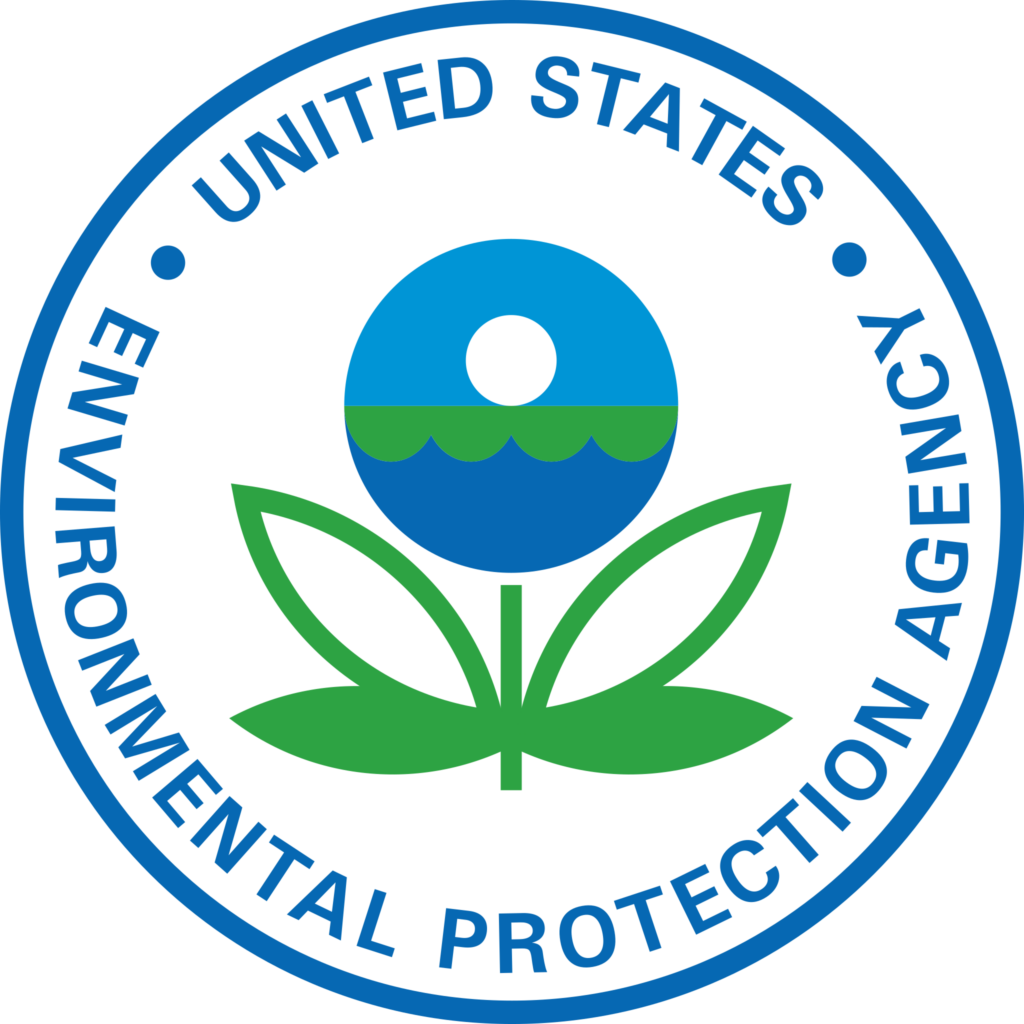
AERMOD (Zou et al., 2010): is an atmospheric dispersion modeling system developed by the American Meteorological Society (AMS)/United States Environmental Protection Agency (EPA) Regulatory Model Improvement Committee. It is the reference model for studying air quality in the United States of America and used worldwide. In a simplified way, AERMOD and other associated tools process terrain/surface data, meteorological data and emission source data, and produce as output the dispersion of emissions in the atmosphere.
We use Polaris to model and simulate traffic. Then we feed the Polaris output data to Autonomie, which generates fuel/energy consumption and emissions data. Finally, we introduce the emission data into AERMOD together with meteorological data so that their dispersion can be simulated.
References
- Auld et al. POLARIS: Agent-based modeling framework development and implementation for integrated travel demand and network and operations simulations. Transportation Research Part C: Emerging Technologies, v. 64, p. 101–116, 2016.
- Barnes et al. Emissions vs exposure: Increasing injustice from road traffic-related air pollution in the United Kingdom. Transportation Research Part D: Transport and Environment, v. 73, p. 56–66, 2019.
- Cars… Cars, planes, trains: where do CO2 emissions from transport come from? Available at: <https://ourworldindata.org/co2-emissions-from-transport>. Accessed on: 11 aug. 2023.
- Conn et al. Introduction to Derivative-Free Optimization. SIAM, 2009.
- Khreis et al. Urban policy interventions to reduce traffic-related emissions and air pollution: A systematic evidence map. Environment International, v. 172, p. 107805, 2023.
- Kim et al. Autonomie model validation with test data for 2010 Toyota Prius. In: SAE Technical Paper Series. SAE International, 2012.
- Lomborg. Welfare in the 21st century: Increasing development, reducing inequality, the impact of climate change, and the cost of climate policies. Technological Forecasting and Social Change, v. 156, p. 119981, 2020.
- Osorio and Bierlaire. A simulation-based optimization framework for urban transportation problems. Operations Research, v. 61, n. 6, p. 1333–1345, 2013.
- Ritchie et al. CO2 and Greenhouse Gas Emissions. Our World in Data, 2020. Available at: <https://ourworldindata.org/co2-and-greenhouse-gas-emissions>. Accessed on: 11 aug. 2023.
- Souza et al. Mesoscopic traffic flow model for agent-based simulation. Procedia Computer Science, v. 151, p. 858–863, 2019.
- Zou, B. et al. Performance of AERMOD at different time scales. Simulation Modelling Practice and Theory, v. 18, n. 5, p. 612–623, 2010.